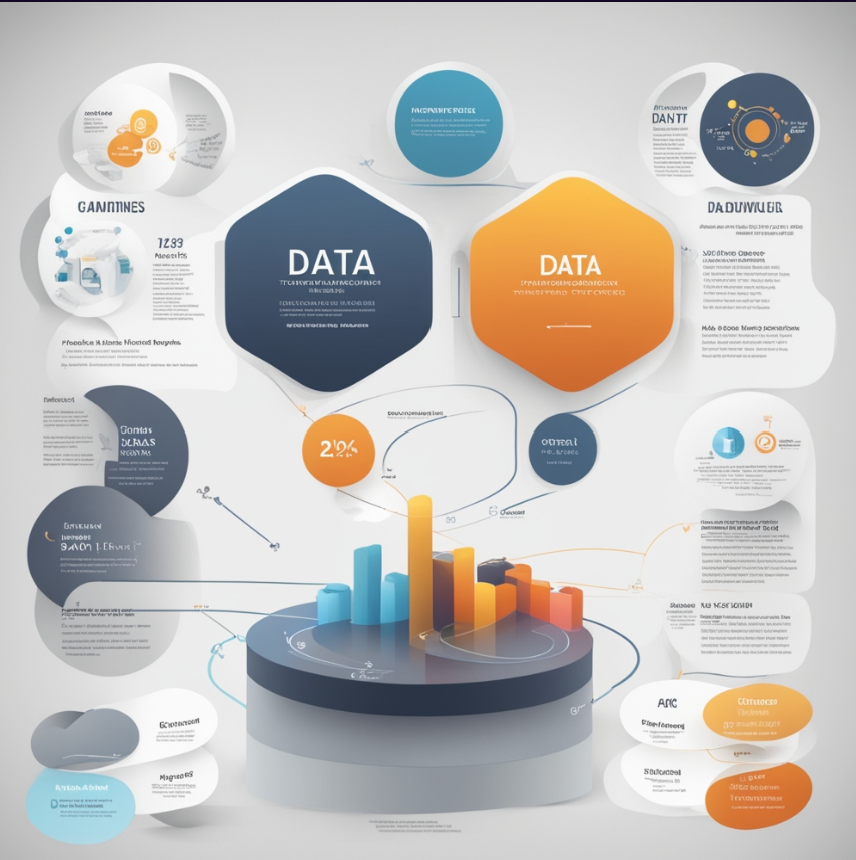
GitHub
Overview
Data transformation analysis involves the process of converting raw data into a structured and usable format for better understanding and decision-making.
This transformative process is crucial in extracting valuable insights from large datasets, enabling organizations to derive meaningful conclusions and make informed decisions.
How it works
In the initial stage, raw data is collected from various sources, such as databases, spreadsheets, or external APIs. The data transformation analysis then begins with data cleaning, where inconsistencies, errors, and missing values are addressed to ensure data accuracy. Subsequently, the data is transformed through techniques such as normalization, aggregation, and filtering, which help in organizing and structuring the data appropriately.
Once the data is prepared, it undergoes analysis to uncover patterns, trends, and correlations. This analysis can involve statistical methods, machine learning algorithms, or other analytical tools depending on the objectives. The insights gained through data transformation analysis play a pivotal role in making data-driven decisions, optimizing business processes, and gaining a competitive edge in various industries. Overall, data transformation analysis is a critical step in the data lifecycle, turning raw information into actionable knowledge.